PhD#2 at Mines Paris in Data Science & Energy: "High-dimensional optimization of distributed assets in smart grids"
ABG-122036 | Thesis topic | |
2024-10-30 | Other public funding |
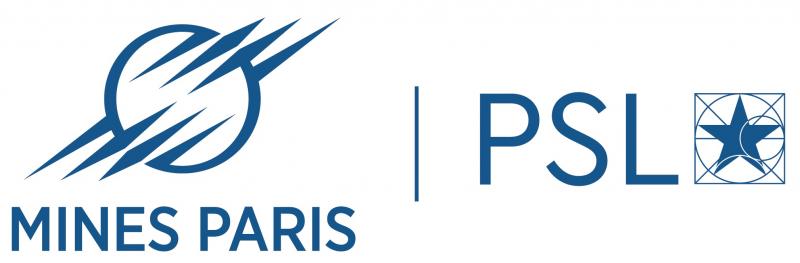
- Engineering sciences
- Energy
- Mathematics
Topic description
Title: "High-dimensional optimization of distributed assets in smart grids"
Context and background:
In the vertically integrated electrical energy systems of the past, power system management was carried out centrally by the transmission and distribution system operators (TSOs, DSOs). In the frame of the energy transition, emerging new actors (aggregators, microgrid operators, energy community managers, self-consumption etc.) and the proliferation of assets connected to the grid, such as renewable energy (RES) plants, storage devices, electric vehicles (EVs), smart-homes/prosumers with IoT devices, electric heating/cooling systems, etc., urge for a paradigm shift towards decentralization. New business models are likely to be based on physical or virtual groupings of assets (“cells”), instantiated as virtual power plants (VPPs), energy communities, microgrids, and others. In the power systems of the future, all these “cell” variants will probably coexist, and their operation should be optimized accounting for the specific interests of the involved actors. For example, a VPP operator aggregates hundreds to thousands of assets to achieve a critical mass of flexibility and valorize it in electricity markets. Optimization functions (“distributed intelligence”) are necessary at these lower levels down to the grid edge (cell, feeders, assets/devices…) and also need to be aligned with the grid operation.
Scientific objectives:
The overarching objective of this research project is to develop distributed optimization methods for grids with a very large number (tens/hundreds of thousands to millions) of connected devices. The aim is to account for the involved uncertainties, the classification of assets/devices into different typologies of virtual/physical cells, their computational/communication capabilities/limitations, environmental disturbances, QoS/grid constraints, and privacy concerns. Large scale distributed optimization requires the design of appropriate grid-aware signals that affect the local optimization processes for a multitude of devices, so that their aggregation provides a predictable response (even though it is the result of the response of different assets), while ensuring an optimal use of grid infrastructure.
Methodology and expected results:
The first step of the research project is a bibliographic research and familiarization with the methods and tools developed at our Group. The initial use case of focus will be the predictive management (scheduling) of the assets (for time frames in the order of a few minutes to a few days ahead). The developed approaches should be scalable to a very high number of assets, with inherent uncertainties in their production/consumption profiles, to cover use cases such as distribution grids and/or VPPs that integrate EVs, RES plants, storage devices, prosumers and the like. The research project will integrate predictive models that reduce the complexity associated to multiple uncertainties, employ machine learning and/or statistical methods for high dimensional problems (e.g., sparse models, functional data analysis, edge ML), and explore distributed optimization strategies to cope with the very large problem sizes. Optimization strategies will involve decomposition methods, blended with machine learning developments (e.g., optimal decision trees able to adapt dynamically to the vast amount of incoming information), and produce signals that account for the local grid conditions (i.e., congestions/overloads) to which the different cells of assets respond based on their capabilities and individual objectives.
Funding category
Funding further details
Presentation of host institution and host laboratory
The PERSEE Center is one of the 18 research centers of MINES Paris. Its field of expertise concerns New Energy Technologies and Renewable Energy Sources (RES). Its research strategy is based on a "micro/macro" approach ranging from (nano)materials to energy systems. It is built around three structuring themes: i) materials and components for energy, ii) sustainable energy conversion and storage processes and technologies, and iii) renewable energies and smart energy systems.
This late is developped by one of the three groups of the Center, ERSEI, which stands for “Renewable Energies and Smart Energy Systems”. The ERSEI group develops methods and tools allowing the optimal integration of decentralized sources, including RES, storage devices, electric vehicles, active demand and other technologies, in energy systems and electricity markets. The research activity of the group is divided into three main themes. The first is based on the development of advanced short-term forecasting methods for different applications in power systems (i.e. forecasting of RES production, demand, dynamic line rating, market quantities, etc.). The second concerns the control and predictive management of energy systems. The aim is to design innovative approaches to optimise the operation (from real-time to days ahead) of different types of systems (smart-homes, microgrids, virtual power plants, energy communities, hybrid RES/storage plants, distribution grids multi-energy systems a.o.) considering uncertainties. The third axis concern planning and prospective studies that aim to optimise the design of future energy systems, generate furture scenarios, optimise investements etc.
The PERSEE Center is located within the scientific parc of Sophia-Antipolis, near the cities of Nice, Cannes and Antibes in the south of France. Its workforce is around 55 people.
Website :
PhD title
Country where you obtained your PhD
Institution awarding doctoral degree
Graduate school
Candidate's profile
Profile:
Engineer and / or Master of Science degree (candidates may apply prior to obtaining their master's degree. The PhD will start though after the degree is succesfully obtained).
Good level of general and scientific culture. Good analytical, synthesis, innovation and communication skills. Qualities of adaptability and creativity. Motivation for research activity. Coherent professional project. Skills in programming (eg R, Python, Julia,…). A succesful candidate will have a solid background in three or more of the following competencies:
- optimization
- applied mathematics, statistics and probabilities
- machine learning, data science
- power systems, renewable integration
Expected level in french : Not required
Expected level in english : Proficiency
Desired starting date: as soon as possible in 2024 or early 2025. Duration 36 months.
Full-time paid position.
For more information please contact Prof. Georges Kariniotakis and Dr. Panagiotis Andrianesis
Vous avez déjà un compte ?
Nouvel utilisateur ?
Get ABG’s monthly newsletters including news, job offers, grants & fellowships and a selection of relevant events…
Discover our members
Tecknowmetrix
ANRT
Institut Sup'biotech de Paris
Nokia Bell Labs France
CESI
SUEZ
ONERA - The French Aerospace Lab
TotalEnergies
Généthon
Aérocentre, Pôle d'excellence régional
ADEME
CASDEN
Laboratoire National de Métrologie et d'Essais - LNE
MabDesign
MabDesign
PhDOOC
Institut de Radioprotection et de Sureté Nucléaire - IRSN - Siège
Ifremer
Groupe AFNOR - Association française de normalisation