Multimodal models for Document Image Understanding
ABG-125039 | Thesis topic | |
2024-07-10 | Other public funding |
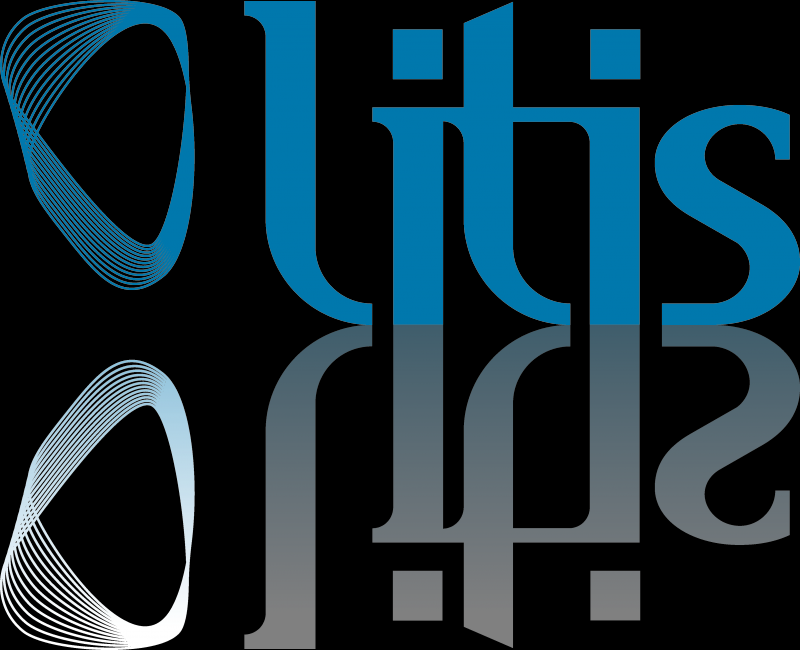
- Computer science
- Data science (storage, security, measurement, analysis)
Topic description
The digital transformation of libraries, which has been based on OCR (Optical Character Recognition) technology for more than 20 years, faces some limitations both in terms of quality, due to the diversity of the collections and the limitations of OCR technology, and in terms of added value due to a lack of structuring and high-level indexing. Named entity extraction is still little used because it relies on language processing technologies, which were not very adaptable until recently. More generally, the semantic indexing of collections is underdeveloped and integrated with metadata. We propose to develop multimodal models (text + image) for the extraction of information from collections of digitized documents in large libraries. The literature shows that work in this direction is still underdeveloped, and that it is mainly aimed at processing commercial documents (invoices etc…).
The proposed project aims to disrupt the traditional sequential document processing workflow by combining Vision models and Large Language Models (LLM) to provide a more streamlined and efficient approach. The standard two-stages architectures based on OCR + NER (Optical Character Recognition, Named Entity Recognition) are now giving way to end-to-end multimodal approaches known as Document Understanding, which are more versatile and easily adaptable to new corpora, making it easier and more cost-effective to set up and run document processing projects. As a result, this accessible, user-friendly approach will democratize access to advanced AI technologies for a wider range of institutions, contributing to the evolution of the technology value chain in the Libraries, Archives and Museums (LAM) sector and opening up new opportunities for research and discovery.
Multimodal Architectures design
A first orientation of the work will aim at integrating into the DAN architecture pre-owned, powerful and royalty-free language representations, such as BERT [6], BART, CAMEMBERT, BLOOM ... Particular attention will be paid to the mode of integration of these representations with regard to their dimension vis-à-vis the dimension of the internal representation of the DAN architecture.
Multimodal architecture training
The integration of language knowledge in the form of a pre-learned model will be considered in different training modalities. Model distillation approaches will be studied. In a more integrated way, we will also try to learn a language representation of the target domain by minimizing the distance between the target representation and the generic representation. In this perspective, one could be inspired by optimal transport approaches. Following the DAN training approach, we aim to explore more in depth using synthetic documents with curriculum learning. One could be inspired by Markovian generative processes or generative adversarial networks (GAN), or diffusion models, to develop an original solution.
Exploring visual question answering (VQA)
One of the most striking developments in recent years is certainly linked to the capacity of large language models to generalize easily from a few examples [7] and without learning, giving rise to specialization through textual interactions with the user (Chat). Even if it seems unthinkable to transpose this type of approach to document understanding, it seems quite relevant to explore the capacity of the architectures we will propose to solve different fictitious or real tasks of visual question answering. We will benefit from some available datasets [8, 9], but we will also explore new scenarios of question-answering tasks in the aim to make the system more adaptive to the user's needs.
Starting date
Funding category
Funding further details
Presentation of host institution and host laboratory
LITIS is a laboratory (UR 4108) of University of Rouen Normandy, University of Havre Normandy and INSA Rouen Normandy. It is a member of the doctoral school MIIS and of the Norman network “Digital Normandy”.
LITIS is a partner of the Normastic CNRS Research Federation.
Research fields : • Information access • Biomedical information processing • Ambient intelligence
Applications : • Health • Automotive, smart territories • Information access in all sectors
Website :
PhD title
Country where you obtained your PhD
Institution awarding doctoral degree
Graduate school
Candidate's profile
We look for a candidate with a curriculum in Machine Learning, and a significant experience of Deep Learning technologies applied to vision or natural language processing (NLP).
Vous avez déjà un compte ?
Nouvel utilisateur ?
Get ABG’s monthly newsletters including news, job offers, grants & fellowships and a selection of relevant events…
Discover our members
Aérocentre, Pôle d'excellence régional
Groupe AFNOR - Association française de normalisation
TotalEnergies
MabDesign
Institut de Radioprotection et de Sureté Nucléaire - IRSN - Siège
PhDOOC
Tecknowmetrix
Laboratoire National de Métrologie et d'Essais - LNE
ONERA - The French Aerospace Lab
MabDesign
Ifremer
Nokia Bell Labs France
ANRT
Institut Sup'biotech de Paris
SUEZ
Généthon
CASDEN
ADEME
CESI
-
JobPermanentRef. ABG123642Laboratoire des Courses Hippiques (GIE LCH)- Ile-de-France - France
Chargé(e) de Recherche et Innovation (H/F) / Senior Scientist Research & Innovation (M/F)
Chemistry - BiochemistryConfirmed -
JobFixed-termRef. ABG125071KTH- Sweden
ERC-funded postdoc position on the detection of gas-phase organic radicals, KTH, Stockholm, Sweden
Chemistry - Physics - Engineering sciencesAny -
JobPermanentRef. ABG124941Corteria Pharmaceuticals- Ile-de-France - France
Jeune Docteur, Chercheur en Biologie Cellulaire & Moléculaire (H/F)
BiologyAny