International PhD Mines Paris/INESC in AI & Energy: "AI-supported stochastic optimization distillation for decision-makers in the energy transition"
ABG-122571 | Sujet de Thèse | |
14/06/2024 | Contrat doctoral |
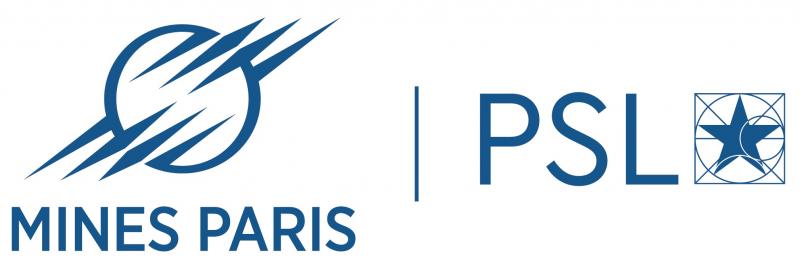
- Sciences de l’ingénieur
- Energie
- Mathématiques
Description du sujet
Title: "AI-supported stochastic optimization distillation for decision-makers in the energy transition"
Context and background:
The stochastic optimization framework has been extensively studied in energy systems, mainly driven to handle the variability and uncertainty of renewable energy sources (RES). Despite the advances in tractability and computational performance of these methods, they are not widely adopted by the industry due to a complex understanding from the decision-maker, which leads to algorithmic aversion. Recently, data-driven prescriptive models (e.g., based on decision trees or reinforcement learning) have been proposed to remove model chain complexity and offer explainability in terms of input features importance, yet they may remain as a “black-box” model to decision-makers and may be negatively affected by data quality. Moreover, in cases where uncertainty is represented by a large number of input scenarios, the interpretability of mathematical optimization models or data-driven techniques is significantly diminished and makes ex-ante and ex-post analysis from humans very complex. An alternative interesting approach in the literature proposes solution sets from the stochastic optimization problem and lets the operators chose the solution according to their priorities and in the region close to the optimum rather than proposing them a hard solution.
Scientific objectives & Methodology:
This Ph.D. topic is inspired by the concept of knowledge distillation (i.e., the process of transferring knowledge from a large model to a smaller one), and the goal is to build reduced or data-driven models that can explain the decisions suggested by stochastic optimization tools. For instance, these models can be trained with data generated by solving multiple instances of the stochastic optimization problem and with several objectives, such as a) explaining how the decision-maker preferences and risk profile affect the optimization output or b) deriving a set of rules that relate input data (e.g., scenarios) and decisions, or c) support the decision-maker in choosing between deterministic and stochastic optimization for a particular context.
Expected results:
The results of this thesis are expected to contribute in increase the acceptability of AI solutions by the different actors of the energy systems. The AI-solutions developed will enhance human capabilities (rather than replacement of human intelligence) in control and trading centers. It will bring to the industry alternative paradigm for accounting for uncertainties in power system management.
International collaboration:
This PhD project, which is supported by the ITN institute or Mines Paris (Institut des Transformations Numériques), is proposed in collaboration with Dr. Ricardo Bessa, Senior Scientist at INESC TEC in Porto who will be PhD co-director. The candidate will pass a period of at least 6 months at INESC TEC. This will permit, among others, to apply for a European Doctorate Label. Additional stays are envisaged to laboratories with related activity to the topic.
INESC TEC is part of the European network HumanE-AI-Net, which aims to develop a new scientific approach to make AI more humanised, consistent with European and universal values and closer to citizens. This network, composed of top European laboratories and researchers in AI, was one of 5 recently approved in the ICT-48 call, and it aims to take an important step in the European AI strategy promoted by the EU, thus consolidating scientific connections in Europe. INESC TEC is also coordinator of the TRUST-AI (“Transparent, Reliable and Unbiased Smart Tool for AI”) project that employs explainable-by-design symbolic models and learning algorithms and adopt a human-centric, guided empirical learning process that integrates cognition. Ricardo Bessa is also coordinator of the EU project AI4REALNET ("AI for real-world network operation).
Prise de fonction :
Nature du financement
Précisions sur le financement
Présentation établissement et labo d'accueil
The PERSEE Center is one of the 18 research centers of MINES Paris. Its field of expertise concerns New Energy Technologies and Renewable Energy Sources (RES). Its research strategy is based on a "micro/macro" approach ranging from (nano)materials to energy systems. It is built around three structuring themes: i) materials and components for energy, ii) sustainable energy conversion and storage processes and technologies, and iii) renewable energies and smart energy systems.
This late is developped by one of the three groups of the Center, ERSEI, which stands for “Renewable Energies and Smart Energy Systems”. The ERSEI group develops methods and tools allowing the optimal integration of decentralized sources, including RES, storage devices, electric vehicles, active demand and other technologies, in energy systems and electricity markets. The research activity of the group is divided into three main themes. The first is based on the development of advanced short-term forecasting methods for different applications in power systems (i.e. forecasting of RES production, demand, dynamic line rating, market quantities, etc.). The second concerns the control and predictive management of energy systems. The aim is to design innovative approaches to optimise the operation (from real-time to days ahead) of different types of systems (smart-homes, microgrids, virtual power plants, energy communities, hybrid RES/storage plants, distribution grids multi-energy systems a.o.) considering uncertainties. The third axis concern planning and prospective studies that aim to optimise the design of future energy systems, generate furture scenarios, optimise investements etc.
PERSEE participates in different projects involving AI and coordinated the H2020 Smart4RES on RES forecasting and applications that involves data science techniques. PERSE is also involved in the PEPR TASE projects AI.NRGY with complementary problematics to the proposed project as well as to Fine4CAST, where it develops energy forecasting methods based on AI.
The PERSEE Center is located within the scientific parc of Sophia-Antipolis, near the cities of Nice, Cannes and Antibes in the south of France. Its workforce is around 55 people.
Site web :
Intitulé du doctorat
Pays d'obtention du doctorat
Etablissement délivrant le doctorat
Ecole doctorale
Profil du candidat
PROFILE:
Engineer and / or Master of Science degree (candidates may apply prior to obtaining their master's degree. The PhD will start though after the degree is succesfully obtained).
Good level of general and scientific culture. Good analytical, synthesis, innovation and communication skills. Qualities of adaptability and creativity. Motivation for research activity. Coherent professional project. Skills in programming (eg,Python, R, Julia,…) and knowledge of optimization tools (e.g, Gurobi, CPLEX). A succesful candidate will have a solid background in two or more of the following competencies:
- optimisation
- applied mathematics, statistics and probabilities
- data science, machine learning, artificial intelligence
- power system management, integration of renewables
Expected level in french : Not required
Expected level in english : Proficiency
Desired starting date : Fall 2024. Duration 36 months. Full-time paid position.
For more information and applications please contact Prof. Georges Kariniotakis and Dr. Simon Camal
Vous avez déjà un compte ?
Nouvel utilisateur ?
Vous souhaitez recevoir nos infolettres ?
-
Sujet de ThèseRef. ABG124643EDFNimes / Chatou - Occitanie - France
Thèse CIFRE sur l'analyse en temps réel des conséquences thermomécaniques du soudage sur les tuyauteries F/H
Sciences de l’ingénieur - Matériaux -
EmploiCDIRef. ABG124374Inn'PulseMassy Palaiseau / Paris 15ème - Ile-de-France - France
Jeune Docteur, Chef/fe de projet R et D électronique
ElectroniqueNiveau d'expérience indifférent