(CIFRE) Optimization of multi-actor energy systems enhanced with AI and privacy-preserving data sharing.
ABG-122602 | Sujet de Thèse | |
30/10/2024 | Autre financement privé |
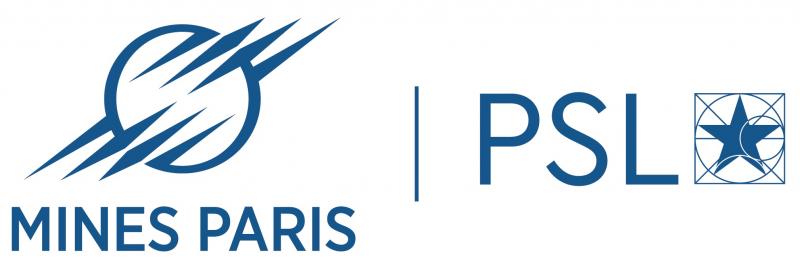
- Sciences de l’ingénieur
- Energie
- Mathématiques
Description du sujet
Title: "Optimization of multi-actor energy systems enhanced with AI and privacy-preserving data sharing".
Context and background:
The energy transition over the next decades is characterized by the development of clean energy sources like renewables (RES), which drive the transition from the centralized energy system towards a decentralized one, where new paradigms for the organization of actors emerge. As an example, we can mention the paradigm of industrial basins with industries that access common resources (i.e. electricity and gas networks), energy communities, microgrids, territories with sector coupling (i.e. gas, e-mobility, district heating). In these paradigms, it is expected that collaborative behavior of the actors, based on the assumption that exchange of information may take place, may permit to reach easier a common objective, like the decarbonization of the industry or the transition to a net-zero territory. From a simple example in the literature we know that, when forecasting the power output of a wind farm, accuracy in the next 6 hours can be improved by up to 20% if data from neighbor wind farms are used as input. However, in practice, data sharing may be hindered due to regulation or commercial/industrial security constraints. Data often comes from smart meters and is therefore subject to confidentiality constraints, or it comes from industrial or renewable energy installations and is therefore commercially sensitive and the corresponding players are reluctant to share it. The obligation to publish information as open data in different sectors does not solve the problem; often, open data is of little value for operational purposes because it is published in aggregate form to mask confidentiality. These constraints limit the value we can extract from available data. Without data sharing, optimization of the energy systems mentioned above leads to sub-optimal solutions.
Scientific objectives and Methodology:
The development of distributed optimization methods, automated transactive peer-to-peer and privacy preserving algorithms offer technical solutions in the field of artificial intelligence that can resolve the above-mentioned bottlenecks and promote collaborative strategies towards common goals like the reduction of the global carbon footprint in highly integrated basins.
The overarching objective of this thesis is double fold:
- to develop methods that permit data sharing, while respecting confidentiality constraints and for different types of data.
- to develop AI-based agents that can support decision-making in multi-actor and collaborative energy schemes and are compatible with privacy-preserving data sharing.
Priority will be given to the use case of an industrial basin. Examples of energy intensive industrial basins in Europe exist, where a single basin represents up to 20% of the country's CO2 emissions. In the process of decarbonization of the involved industries, data sharing can be critical. Key themes and questions proposed in this topic are along the following lines:
- How can automated negotiation technology provided by peer to peer exchange design can support coordination between industrial agents in a basin?
- Can decomposition methods be used to coordinate heterogeneous optimization problems to reach a common goal without sharing of the underlying data and models.
- How can privacy-preserving learning provide a collaborative modelling framework for the actors of an industrial basin to improve the global modelling accuracy with no or limited data sharing?
Expected results:
Although the applicative field in this PhD project is energy systems, the techniques developed have a replication potential to other fields like health or other. The expected results contain:
- Algorithms for data sharing, while preserving confidentiality constraints.
- Distributed optimization methods that implement data sharing and AI techniques.
- Evaluation on real data with focus to use cases of industrial basins.
- Recommendations to regulatory authorities and policy makers.
International collaboration:
The candidate will pass a period of at least one 6 months at Imperial College in London in the team of the PhD co-director Prof. Pierre PINSON. This will permit, among others, to apply for a European Doctorate Label. Additional stays are possible at laboratories with related activity to the topic like INESC TEC in Porto. Non-academic stays are foreseen at the R&D center of Air-Liquide in Paris area.
Nature du financement
Précisions sur le financement
Présentation établissement et labo d'accueil
The PERSEE Center is one of the 18 research centers of MINES Paris. Its field of expertise concerns New Energy Technologies and Renewable Energy Sources (RES). Its research strategy is based on a "micro/macro" approach ranging from (nano)materials to energy systems. It is built around three structuring themes: i) materials and components for energy, ii) sustainable energy conversion and storage processes and technologies, and iii) renewable energies and smart energy systems.
This late is developped by one of the three groups of the Center, ERSEI, which stands for “Renewable Energies and Smart Energy Systems”. The ERSEI group develops methods and tools allowing the optimal integration of decentralized sources, including RES, storage devices, electric vehicles, active demand and other technologies, in energy systems and electricity markets. The research activity of the group is divided into three main themes. The first is based on the development of advanced short-term forecasting methods for different applications in power systems (i.e. forecasting of RES production, demand, dynamic line rating, market quantities, etc.). The second concerns the control and predictive management of energy systems. The aim is to design innovative approaches to optimise the operation (from real-time to days ahead) of different types of systems (smart-homes, microgrids, virtual power plants, energy communities, hybrid RES/storage plants, distribution grids multi-energy systems a.o.) considering uncertainties. The third axis concern planning and prospective studies that aim to optimise the design of future energy systems, generate furture scenarios, optimise investements etc.
PERSEE participates in different projects involving AI and coordinated the H2020 Smart4RES on RES forecasting and applications that involves data sharing techniques. PERSE is also involved in the PEPR TASE projects AI.NRGY with complementary problematics on privacy-preserving data sharing.
The PERSEE Center is located within the scientific parc of Sophia-Antipolis, near the cities of Nice, Cannes and Antibes in the south of France. Its workforce is around 55 persons.
Site web :
Intitulé du doctorat
Pays d'obtention du doctorat
Etablissement délivrant le doctorat
Ecole doctorale
Profil du candidat
REQUESTED PROFILE:
Engineer and / or Master of Science degree (candidates may apply prior to obtaining their master's degree. The PhD will start though after the degree is succesfully obtained). Good level of general and scientific culture. Good analytical, synthesis, innovation and communication skills. Qualities of adaptability and creativity. Motivation for research activity. Coherent professional project.
Specific skills for this thesis: A successful candidate will have a strong background in applied mathematics (i.e. optimization) and/or AI and data science.
Desired backround in energy engineering.
Skills in computer programming (i.e. Python, R, Julia) are required together with knowledge of optimization tools (e.g, Gurobi, CPLEX).
Expected level in French: Not required
Expected level in English: Proficiency
Vous avez déjà un compte ?
Nouvel utilisateur ?
Vous souhaitez recevoir nos infolettres ?
Découvrez nos adhérents
ONERA - The French Aerospace Lab
Nokia Bell Labs France
ADEME
MabDesign
Institut de Radioprotection et de Sureté Nucléaire - IRSN - Siège
MabDesign
CASDEN
Institut Sup'biotech de Paris
Tecknowmetrix
Généthon
Groupe AFNOR - Association française de normalisation
SUEZ
PhDOOC
Ifremer
CESI
TotalEnergies
Laboratoire National de Métrologie et d'Essais - LNE
Aérocentre, Pôle d'excellence régional
ANRT