Quantifying Uncertainty in Machine Learning Interatomic Potentials for Accurate Molecular Dynamics Simulations
ABG-128311 | Sujet de Thèse | |
03/02/2025 | Contrat doctoral |
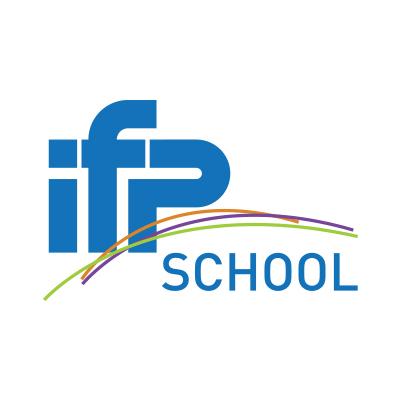
- Mathématiques
Description du sujet
In the pursuit of energy transition, optimizing catalytic processes is essential for the efficient and sustainable conversion of raw materials into biofuels. At the heart of this optimization lies a deep understanding of atomic-level reaction mechanisms. Molecular Dynamics (MD) simulations, coupled with quantum mechanical calculations (DFT), provide powerful insights into these mechanisms. Yet, the high computational cost of DFT presents a significant challenge.
To address this limitation, Machine Learning (ML) approaches have emerged as a promising solution, accelerating simulations by replicating DFT results at a fraction of the computational cost using Machine Learning Interatomic Potentials (MLIP). Despite their advantages, MLIP models are prone to accumulating errors during simulations, which can undermine the reliability of the results. This PhD aims to develop a robust uncertainty quantification framework to control and estimate errors in MLIP-based Molecular Dynamics simulations, enhancing the reliability of reaction rate predictions.
• Error Control Framework: Design an uncertainty quantification method that triggers DFT calculations when MLIP errors exceed a predefined threshold.
• Error Propagation in Kinetics: Develop techniques to propagate uncertainties through MD simulations, enabling accurate estimation of reaction rate constants.
• Extension to Nonlinear Models: Adapt the uncertainty quantification framework for nonlinear MLIP models, such as graph neural networks, to broaden its applicability to complex interatomic potentials.
The proposed methods will be validated using the dehydration of isobutanol catalyzed by acidic zeolites—a reaction of significant interest in biomass conversion due to its complexity and industrial relevance.
Prise de fonction :
Nature du financement
Précisions sur le financement
Présentation établissement et labo d'accueil
IFP Energies nouvelles is a French public-sector research, innovation and training center. Its mission is to develop efficient, economical, clean and sustainable technologies in the fields of energy, transport and the environment. For more information, see our WEB site.
IFPEN offers a stimulating research environment, with access to first in class laboratory infrastructures and computing facilities. IFPEN offers competitive salary and benefits packages. All PhD students have access to dedicated seminars and training sessions.
Site web :
Profil du candidat
- Academic requirements University Master or Engineering School degree in applied mathematics, statistics/probability, machine learning
- Language requirements English level B2 (CEFR), willingness to learn French
- Other requirements solid knowledge in programming (Python), curiosity and critical thinking skills
Vous avez déjà un compte ?
Nouvel utilisateur ?
Vous souhaitez recevoir nos infolettres ?
Découvrez nos adhérents
MabDesign
Nokia Bell Labs France
Institut Sup'biotech de Paris
CASDEN
Généthon
Ifremer
MabDesign
Institut de Radioprotection et de Sureté Nucléaire - IRSN - Siège
Tecknowmetrix
SUEZ
ADEME
TotalEnergies
ANRT
Laboratoire National de Métrologie et d'Essais - LNE
PhDOOC
CESI
Groupe AFNOR - Association française de normalisation
Aérocentre, Pôle d'excellence régional
ONERA - The French Aerospace Lab