PhD CIFRE RTE - MINES PARIS: Artificial Intelligence for Renewable Energy Forecasting
ABG-131449 | Sujet de Thèse | |
25/04/2025 | Cifre |
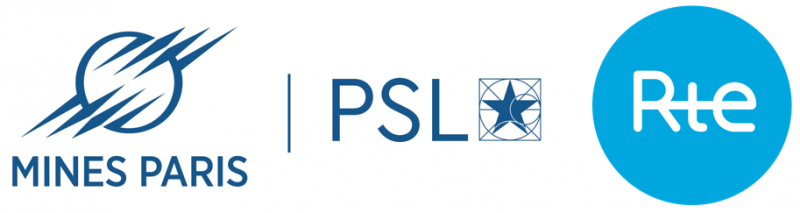
- Sciences de l’ingénieur
- Energie
- Mathématiques
Description du sujet
Title: "Advanced AI-based methods to exploit massive data for improved predictability of renewable energy generation. "
Context and background:
Short-term energy forecasting for the next minutes to days ahead, is a prerequisite for the economic and safe operation of modern power systems and electricity markets, especially under high penetration of renewable energy sources (RES). Given the diverse application contexts, end-users require models that have a wide range of properties especially when deployed in operational settings.
Although RES forecasting models have been used operationally for years, numerous challenges remain unresolved, continuing to drive research efforts worldwide. This PhD project focuses on RES forecasting across various geographical scales: local, regional, and national. Improving forecast accuracy is a more cost-effective strategy that permits to reduce investments in hedging solutions such as storage.
At time scales of a few minutes to a few hours ahead, there is a significant potential for improving accuracy by leveraging data from geographically distributed RES plants. These plants can act as sensors, capturing the propagation of weather phenomena in space and time. This information can then be used to produce more precise predictions for individual RES sites.
The growing amount of installed RES plants results in massive amounts of data that have to be efficiently processed by the forecasting models. Additionally, data may be missing or affected by non-weather-related factors. For example, wind turbines might be slowed down due to noise reduction regulations, or may be stopped to avoid bird collision or due to electricity grid constraints.
Forecasts must also maintain consistency across different geographical levels; from individual wind farms to regional and national scale (i.e. wind farm/region/national). At present, RTE, the French Transmission System Operator, uses RES forecasts for the short-term balancing of electricity supply and demand and to estimate the conditions at the electricity grid (power flow). Even a few percentages of error in RES production at the national scale are translated to very high financial costs (several millions euros per day) and may jeopardise the grid operator’s obligation to ensure security of supply. Therefore, improving RES forecasting accuracy is a top priority for grid operators (https://www.rte-france.com/en/uninterrupted-flow-current/balancing-supply-and-demand). Modern IA-based methods offer a great potential towards that direction.
Scientific objectives:
The aim of this thesis is to improve predictability of RES production and net load, with a focus on challenging situations due to extreme weather conditions, missing or corrupted data and non-weather-related factors that affect RES production. The research will explore the application of AI-based methodologies, including foundational models, deep learning architectures, and more conventional AI approaches as reference, to tackle these challenges. A core objective is to assess the ability of such models to process large-scale datasets, integrate contextual information, and adapt to changing system configurations (i.e. evolution in the number of installed RES plants). Furthermore, the thesis aims to develop forecasting models capable of generating consistent prediction across different geographical scales.
Methodology and expected results:
Initially the state of the art will be analysed.Given the vast volume of existing literature—often characterized by incremental advancements—this task presents a significant challenge. To efficiently identify high-potential innovations, a smart and possibly AI-assisted approach will be developed to filter, classify, and extract the most impactful contributions. The integration of additional data sources, such as alternative Numerical Weather Predictions (NWPs), radar observations, and data from meteorological stations, will also be explored to enhance forecast performance.
Benchmarks will be established based on existing forecasts used by RTE, as well as state-of-the-art methods. The prediction errors will be analyzed in relation to input data, weather conditions, and other contextual variables, in order to identify situations more prone to improvements. Building on these insights, novel forecasting approaches will be proposed with a focus on the spatiotemporal approach and/or AI-based approaches. The aim is to develop forecasting solutions that are not only more accurate but also more resilient and context-aware.
Prise de fonction :
Nature du financement
Précisions sur le financement
Présentation établissement et labo d'accueil
- MINES PARIS - PSL, Centre PERSEE
The PERSEE Center is one of the 18 research centers of MINES Paris. Its field of expertise concerns New Energy Technologies and Renewable Energy Sources (RES). Its research strategy is based on a "micro/macro" approach ranging from (nano)materials to energy systems. It is built around three structuring themes: i) materials and components for energy, ii) sustainable energy conversion and storage processes and technologies, and iii) renewable energies and smart energy systems.
This late is developped by one of the three groups of the Center, ERSEI, which stands for “Renewable Energies and Smart Energy Systems”. The ERSEI group develops methods and tools allowing the optimal integration of decentralized sources, including RES, storage devices, electric vehicles, active demand and other technologies, in energy systems and electricity markets. The research activity of the group is developped through three main axes. The first is based on the development of advanced short-term forecasting methods for different applications in power systems (i.e. forecasting of RES production, demand, dynamic line rating, market quantities, etc.). The second concerns the control and predictive management of energy systems. The aim is to design innovative approaches to optimise the operation (from real-time to days ahead) of different types of systems (smart-homes, microgrids, virtual power plants, energy communities, hybrid RES/storage plants, distribution grids multi-energy systems a.o.) considering uncertainties. The third axis concern planning and prospective studies that aim to optimise the design of future energy systems, generate furture scenarios, optimise investements etc.
The PERSEE Center is located within the scientific parc of Sophia-Antipolis, near the cities of Nice, Cannes and Antibes in the south of France. Its workforce is around 55 people.
- RTE (Réseau de Transport d’Électricité)
RTE is the French electricity transmission system operator. It is responsible for managing and maintaining the high-voltage electricity network in France, which represents more than 100,000 km of transmission lines and numerous substations. RTE ensures a continuous and balanced supply of electricity across France while ensuring interconnection with the neighboring countries. RTE plays a crucial role in the energy transition by adapting the network to facilitate the increasing integration of renewable energy sources and by contributing significantly to the decarbonization of the French electrical system. Furthermore, the company provides data, forecasts, and expert analyses related to the energy system.
Site web :
Intitulé du doctorat
Pays d'obtention du doctorat
Etablissement délivrant le doctorat
Ecole doctorale
Profil du candidat
Engineer and / or Master of Science degree (candidates may apply prior to obtaining their master's degree. The PhD will start though after the degree is successfully obtained).
Good level of general and scientific culture. Good analytical, synthesis, innovation and communication skills. Qualities of adaptability and creativity. Motivation for research activity. Coherent professional project. Skills in programming (eg. Python,…).
A successful candidate will have a solid background in two or more of the following competencies:
- applied mathematics, statistics and probabilities
- data science, machine learning, artificial intelligence
- energy forecasting
Expected level in french : good level recommended
Expected level in english : excellent
Vous avez déjà un compte ?
Nouvel utilisateur ?
Vous souhaitez recevoir nos infolettres ?
Découvrez nos adhérents
ONERA - The French Aerospace Lab
CESI
ANRT
Tecknowmetrix
ADEME
Aérocentre, Pôle d'excellence régional
SUEZ
Institut Sup'biotech de Paris
Laboratoire National de Métrologie et d'Essais - LNE
ASNR - Autorité de sûreté nucléaire et de radioprotection - Siège
TotalEnergies
MabDesign
Groupe AFNOR - Association française de normalisation
Ifremer
Nokia Bell Labs France
Généthon
PhDOOC
MabDesign
CASDEN