Impedance Based Temperature Estimation of LithiumSulfur Cells Using Artificial Neural Networks.
ABG-129522 | Sujet de Thèse | |
14/03/2025 | Contrat doctoral |
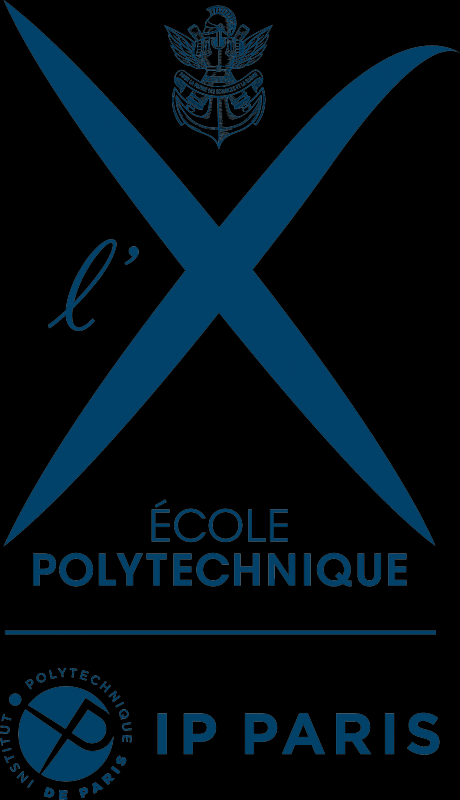
- Numérique
- Science de la donnée (stockage, sécurité, mesure, analyse)
Description du sujet
Among various energy storage technologies, rechargeable batteries, particularly lithium-ion batteries (LiBs) are widely used as power sources due to their advantages as high operating voltage and current, high energy density, light weight, long cycle life, and environmental friendliness.[1] However, the maximum theoretical energy density of commercial LiBs remains insufficient to meet the demands of future energy-intensive technologies. Additionally, most research and development efforts have focused on room-temperature applications. The performance of LiBs is highly dependent on cell temperature, particularly in relation to battery aging and safety issues.[2] At low temperatures, reduced reaction kinetics increase the risk of lithium plating, leading to decreased lithium availability. Conversely, high temperatures can cause undesirable side reactions, resulting in rapid degradation. Furthermore, excessive temperatures may cause material decomposition, triggering thermal runaway, which can lead to an explosion.[2] To address these challenges, future battery technologies must maintain optimal performance across a wide temperature range to meet demands of extreme conditions. Recently, considerable efforts have been devoted to developing extended range (low-, high-) temperature energy storage systems, with lithium-sulfur (Li-S) batteries emerging as one of the most promising candidates. Li-S batteries offer a theoretical specific energy of 2600 Wh kg-1 , approximately five times higher than the current standard for LiBs. Furthermore, sulfur is abundant, affordable, and non-toxic. In addition, Li-S batteries exhibit high energy density under low-temperature conditions, making them particularly suitable for extreme environments.[3] Impedance-based methods have gained significant interest due to their ability to estimate the average internal temperature of a battery without requiring additional internal or external hardware. As a result, these methods are often referred to as sensorless temperature measurement techniques.[4] This thesis explores a sensorless temperature estimation method that combines data from electrochemical impedance spectroscopy (EIS) with artificial neural networks (ANNs). [4] Traditionally, a Battery Management System (BMS) relies on integrated sensors, such as thermistors or thermocouples, to monitor internal temperature and to prevent hazards like thermal runaway.[5] In sensorless methods, the impedance spectrum of a battery, obtained through EIS, contains subtle signatures that correlate with its internal temperature. By training an ANN to recognize these patterns, it becomes possible to predict the battery’s internal temperature using measurable electrical signals (voltage, current, and time). When integrated into an advanced BMS, this approach enables real-time thermal monitoring and management, enhancing safety and mitigating risks such as overheating, fires, or explosions. A key advantage of the EIS-ANN method is its rapid temperature estimation, which is completed within milliseconds, eliminating the need for computationally intensive partial differential equation solving.
Prise de fonction :
Nature du financement
Précisions sur le financement
Présentation établissement et labo d'accueil
The LPICM (Laboratoire de Physique des Interfaces et des Couches Minces) at École Polytechnique focuses on cutting-edge research in the physics of materials, particularly at the nanoscale. The lab conducts research in various fields, including nanostructures, surface physics, and quantum electronics, with applications in photodetection, photovoltaic, polarimetry, energy, sensing, and next-generation technologies.
Intitulé du doctorat
Pays d'obtention du doctorat
Profil du candidat
The candidate should hold a Master 2 Research degree in applied physics, materials science or computer science and have strong experience in AI and machine learning. Proficiency in python, MALAB or similar programming languages for AI model development and data processing is required. knowledge of electrochemical impedance spectroscopy is essential and familiarity with batteries would be an advantage. Excellent written and verbal communication skills (English mandatory) and independent in writing reports. Strong team player. The candidate will have to provide all the diplomas already obtained, as well as a transcript of the marks already acquired for the two years of Master.
Vous avez déjà un compte ?
Nouvel utilisateur ?
Vous souhaitez recevoir nos infolettres ?
Découvrez nos adhérents
Ifremer
Institut Sup'biotech de Paris
Nokia Bell Labs France
Groupe AFNOR - Association française de normalisation
SUEZ
ADEME
ANRT
Aérocentre, Pôle d'excellence régional
Généthon
ASNR - Autorité de sûreté nucléaire et de radioprotection - Siège
MabDesign
CESI
ONERA - The French Aerospace Lab
TotalEnergies
PhDOOC
MabDesign
CASDEN
Tecknowmetrix
Laboratoire National de Métrologie et d'Essais - LNE
-
Sujet de ThèseRef. 130176Strasbourg , Grand Est , FranceInstitut Thématique Interdisciplinaire IRMIA++
Schrödinger type asymptotic model for wave propagation
Expertises scientifiques :Mathématiques - Mathématiques
-
EmploiRef. 130080Paris , Ile-de-France , FranceAgence Nationale de la Recherche
Chargé ou chargée de projets scientifiques bioéconomie H/F
Expertises scientifiques :Biochimie
Niveau d’expérience :Confirmé